Welcome!
I'm a final year Computer Science Ph.D. student at Brown University, where I'm fortunate to be advised by Daniel Ritchie.
I'm interested in developing (structured) learning-based techniques to better understand and represent visual data. Many of my projects involve visual programs (symbolic procedures that output shapes when executed), for instance, how to: infer programs from data, synthesize novel shapes, or automatically discover better domain-specific languages.
Publications
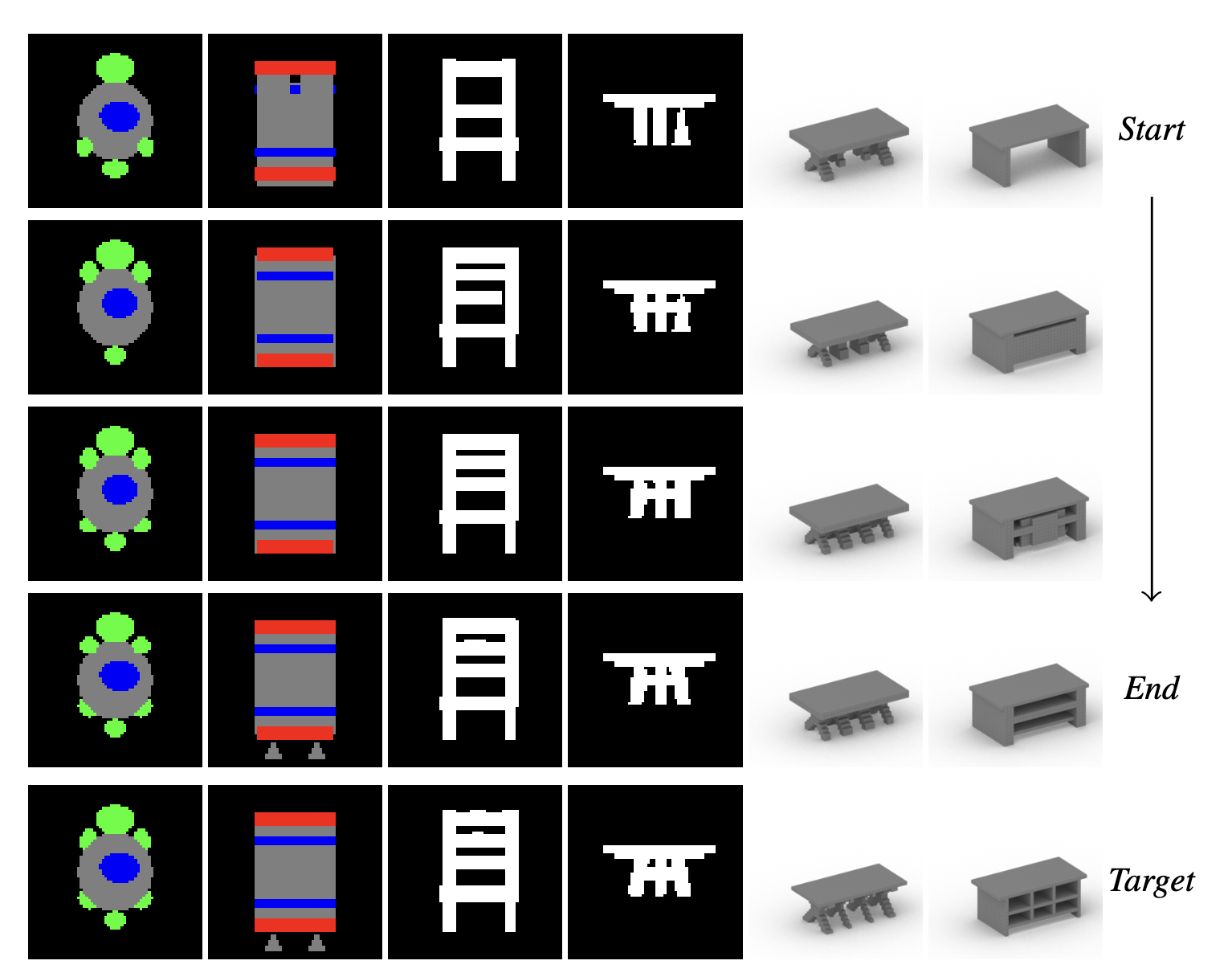
Learning to Edit Visual Programs with Self-Supervision
NeurIPS 2024
Paper
|
Project Page
|
Code
|
Video
We design a system that learns how to edit visual programs. Given an initial program and a visual target, our network predicts local edit operations that can be applied to the input program to improve its similarity to the target.
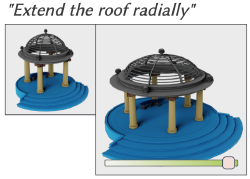
ParSEL: Parameterized Shape Editing with Language
SIGGRAPH Asia 2024
Paper
We introduce ParSEL, a system that enables controllable editing of high-quality 3D assets from natural language. Given a segmented 3D mesh and an editing request, ParSEL produces a parameterized editing program that allows users to explore a family of shape variations with precise controls.
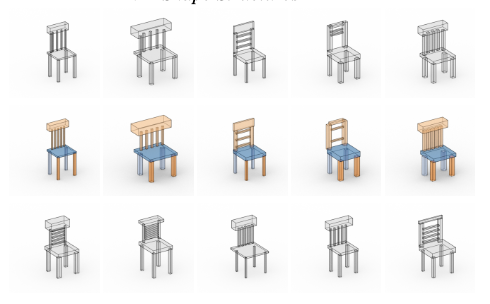
Learning to Infer Generative Template Programs for Visual Concepts
ICML 2024
Paper
|
Project Page
|
Code
We develop a neurosymbolic method that learns how to infer Template Programs; partial programs that capture visual concepts in a domain-general fashion. Our framework supports multiple concept-related tasks: cosegmentation, few-shot generation, and concept synthesis.
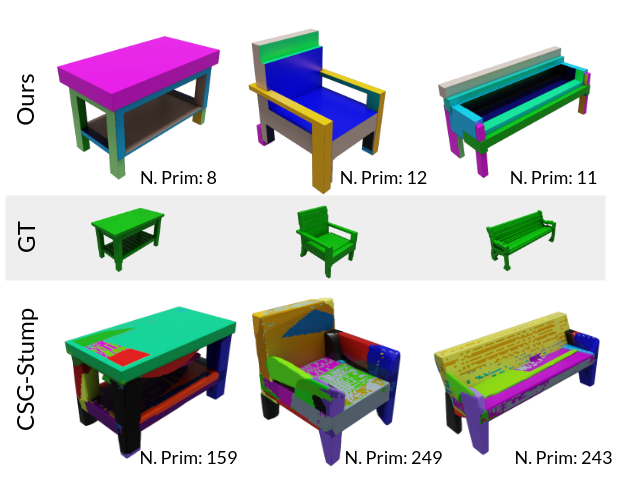
Improving Unsupervised Visual Program Inference with Code Rewriting Families
ICCV 2023
Paper
|
Project Page
|
Code
|
Supplemental
We explore how code rewriting can be used to improve visual program induction networks. Across multiple domains, our family of visual program rewriters treat programs as structured objects to produce better training targets for bootstrapped learning methods.
Oral Presentation
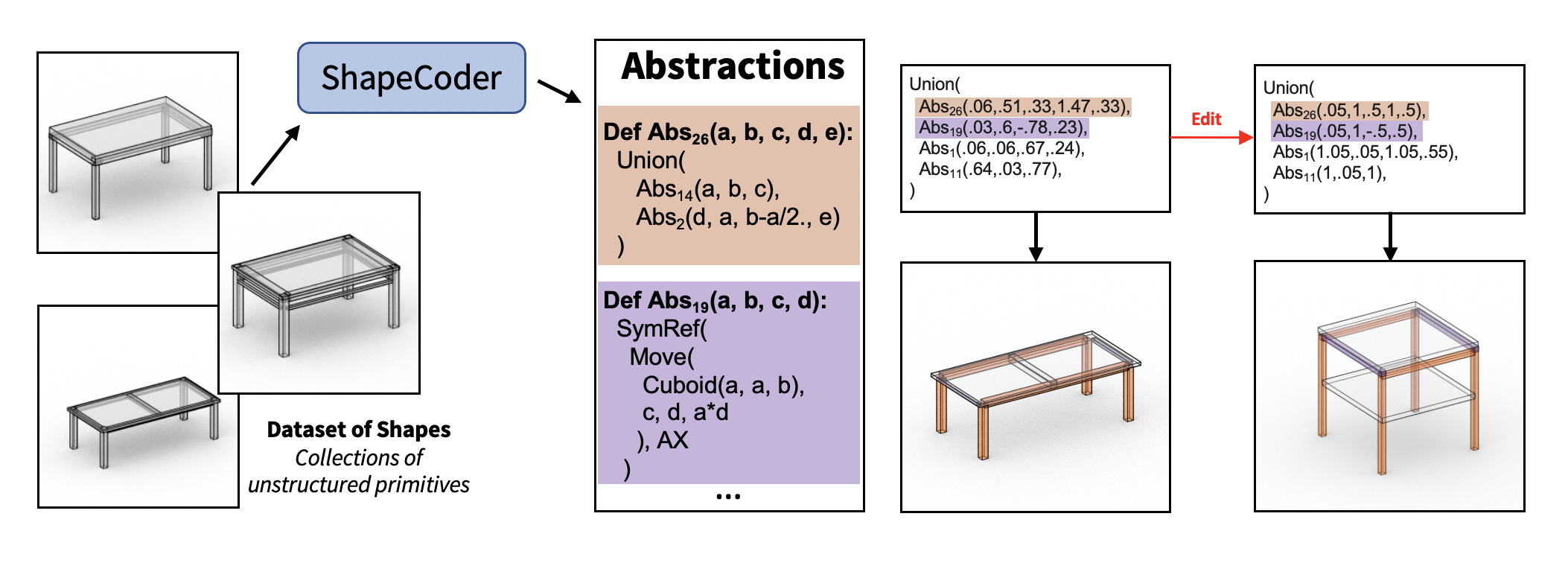
ShapeCoder: Discovering Abstractions for Visual Programs from Unstructured Primitives
SIGGRAPH 2023
Paper
|
Project Page
|
Code
|
Supplemental
ShapeCoder automatically discovers abstraction functions, and infers visual programs that use these abstractions, to compactly explain an input dataset of shapes represented with unstructured primitives. Discovered abstractions capture common patterns (both structural and parametric) so that programs rewritten with these abstractions are more compact and expose fewer degrees of freedom.
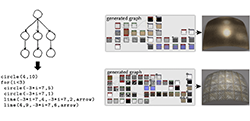
Neurosymbolic Models for Computer Graphics
Eurographics 2023 STAR
Paper
We summarize research on neurosymbolic models in computer graphics: methods that combine the strengths of both AI and symbolic programs to represent, generate, and manipulate visual data.
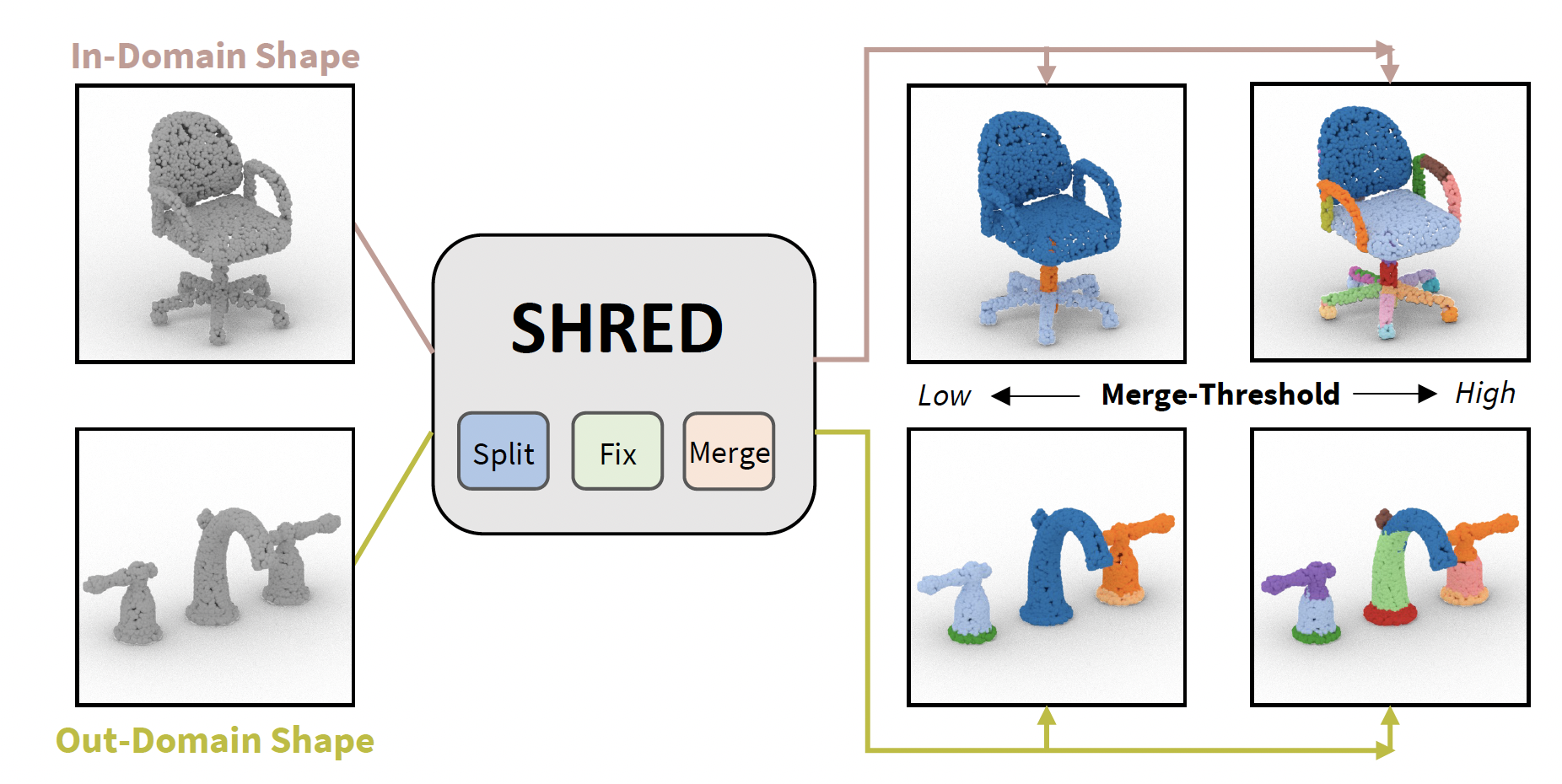
SHRED: 3D Shape Region Decomposition with Learned Local Operations
SIGGRAPH Asia 2022
Paper
|
Project Page
|
Code
|
Video
|
Supplemental
SHRED is a method for 3D SHape REgion Decomposition that consumes a 3D shape as input and uses learned local operations to produce a segmentation that approximates fine-grained part instances.
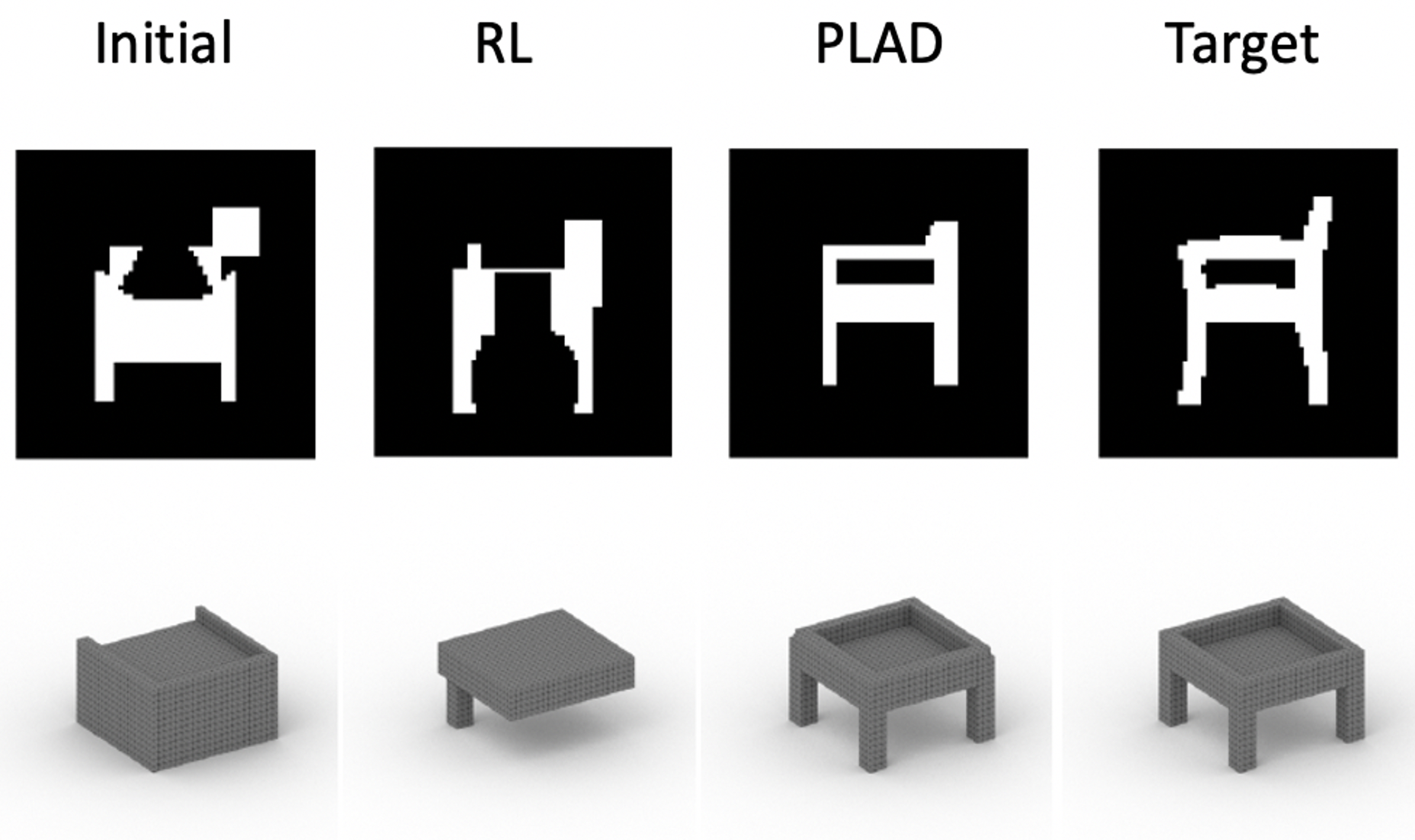
PLAD: Learning to Infer Shape Programs with Pseudo-Labels and Approximate Distributions
CVPR 2022
Paper
|
Project Page
|
Code
|
Video
|
Supplemental
We group a family of shape program inference methods under a single conceptual framework, where training is performed with maximum likelihood updates sourced from either Pseudo-Labels or an Approximate Distribution (PLAD). Compared with policy gradient reinforcement learning, we show that PLAD techniques infer more accurate shape programs and converge significantly faster.
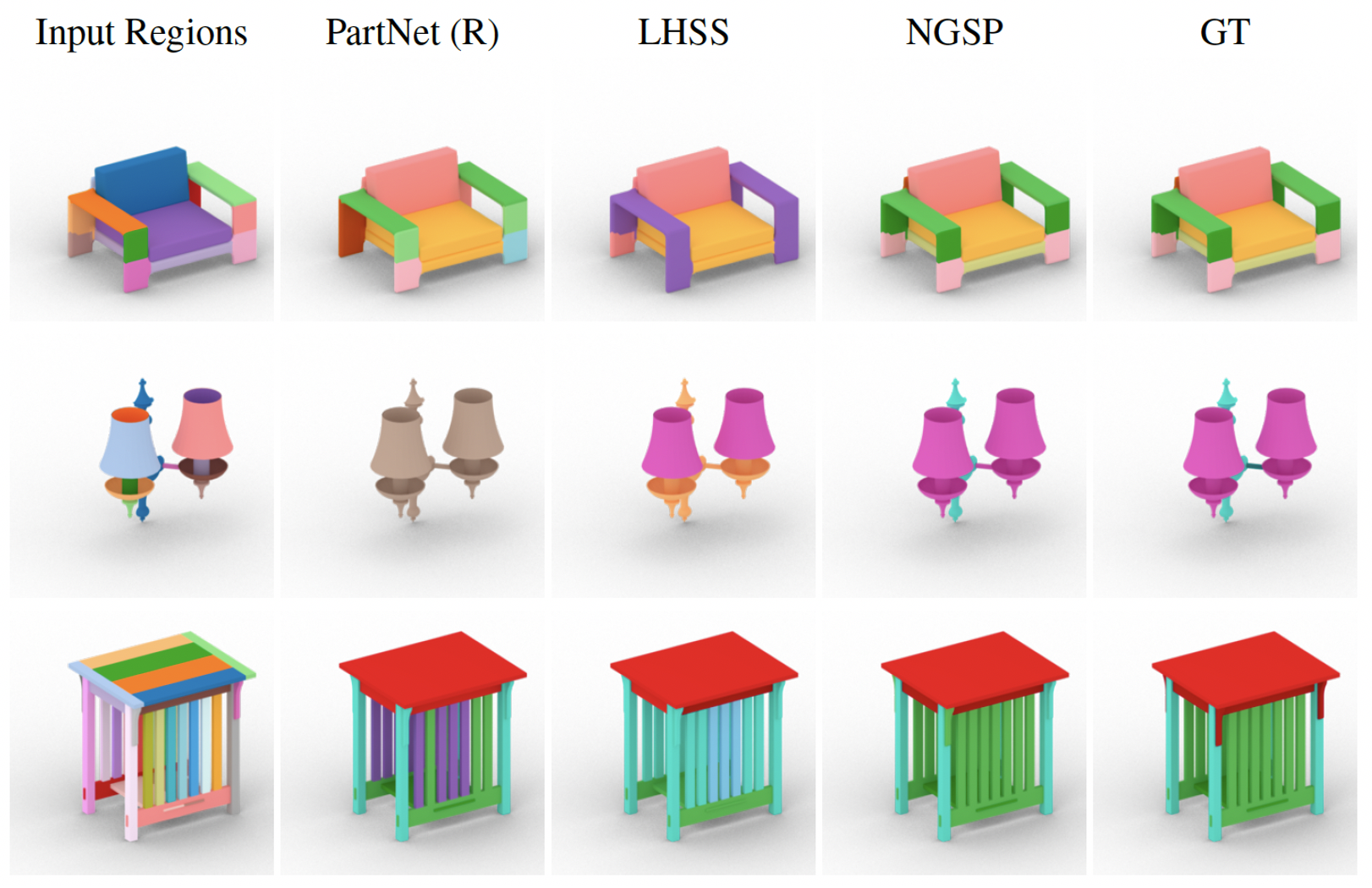
The Neurally-Guided Shape Parser: Grammar-based Labeling of 3D Shape Regions with Approximate Inference
CVPR 2022
Paper
|
Project Page
|
Code
|
Video
|
Supplemental
We frame 3D shape semantic segmentation as a label assignment problem over shape regions; in this paradigm, we show our approximate inference formulation improves performance over comparison methods that (i) use regions to group per-point predictions, (ii) use regions as a self-supervisory signal or (iii) assign labels to regions under alternative formulations.
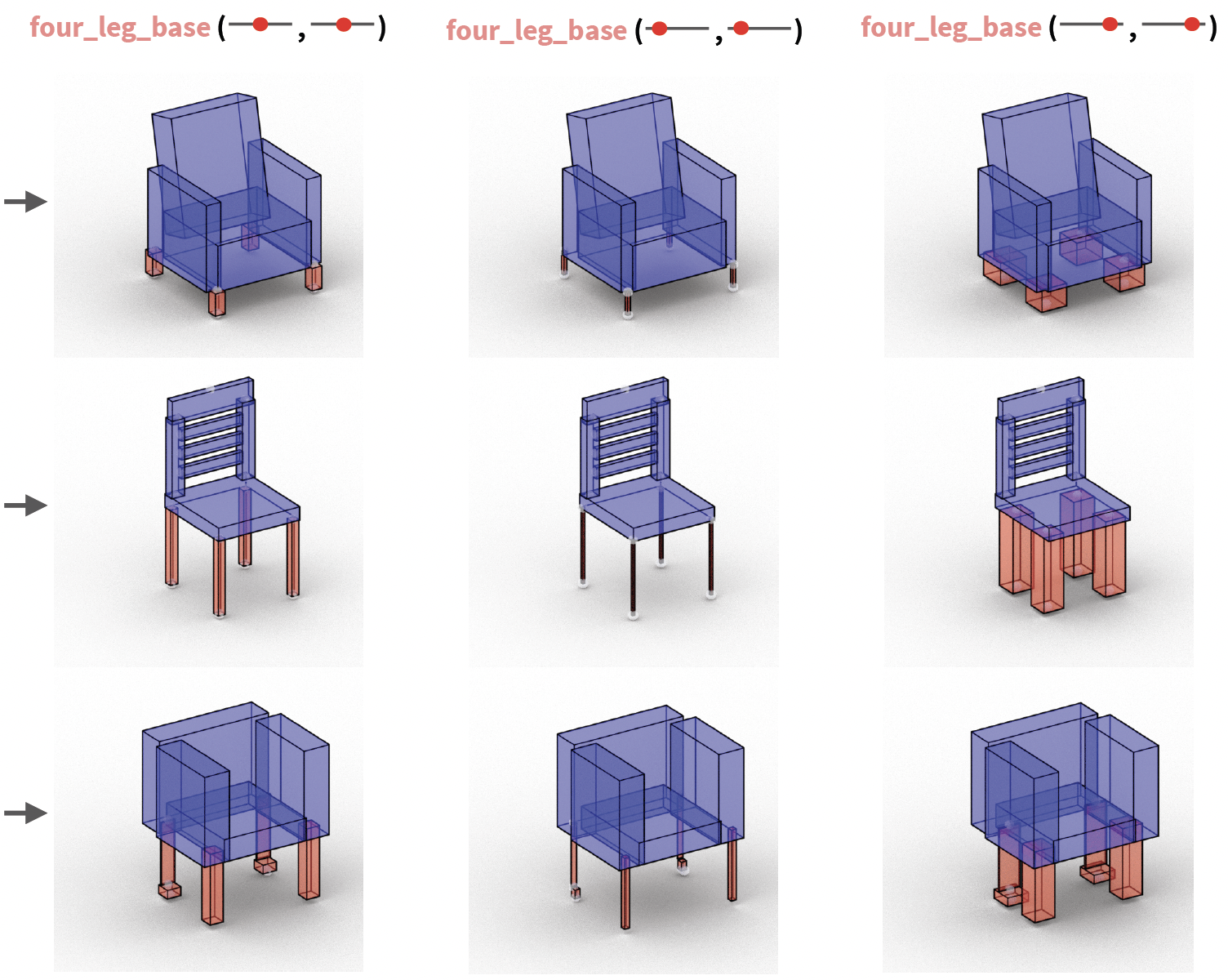
ShapeMOD: Macro Operation Discovery for 3D Shape Programs
SIGGRAPH 2021
Paper
|
Project Page
|
Code
|
Video
|
Supplemental
An algorithm that automatically discovers macro operators that are useful for collections of 3D shape programs. It discovers macros that make programs more compact by minimizing the number of function calls and free parameters required to represent a dataset of imperative programs that may contain continuous parameters. We show these discovered macros improve performance on down-stream tasks such as program inference from unstructured geometry, generative modeling, and goal-directed editing.
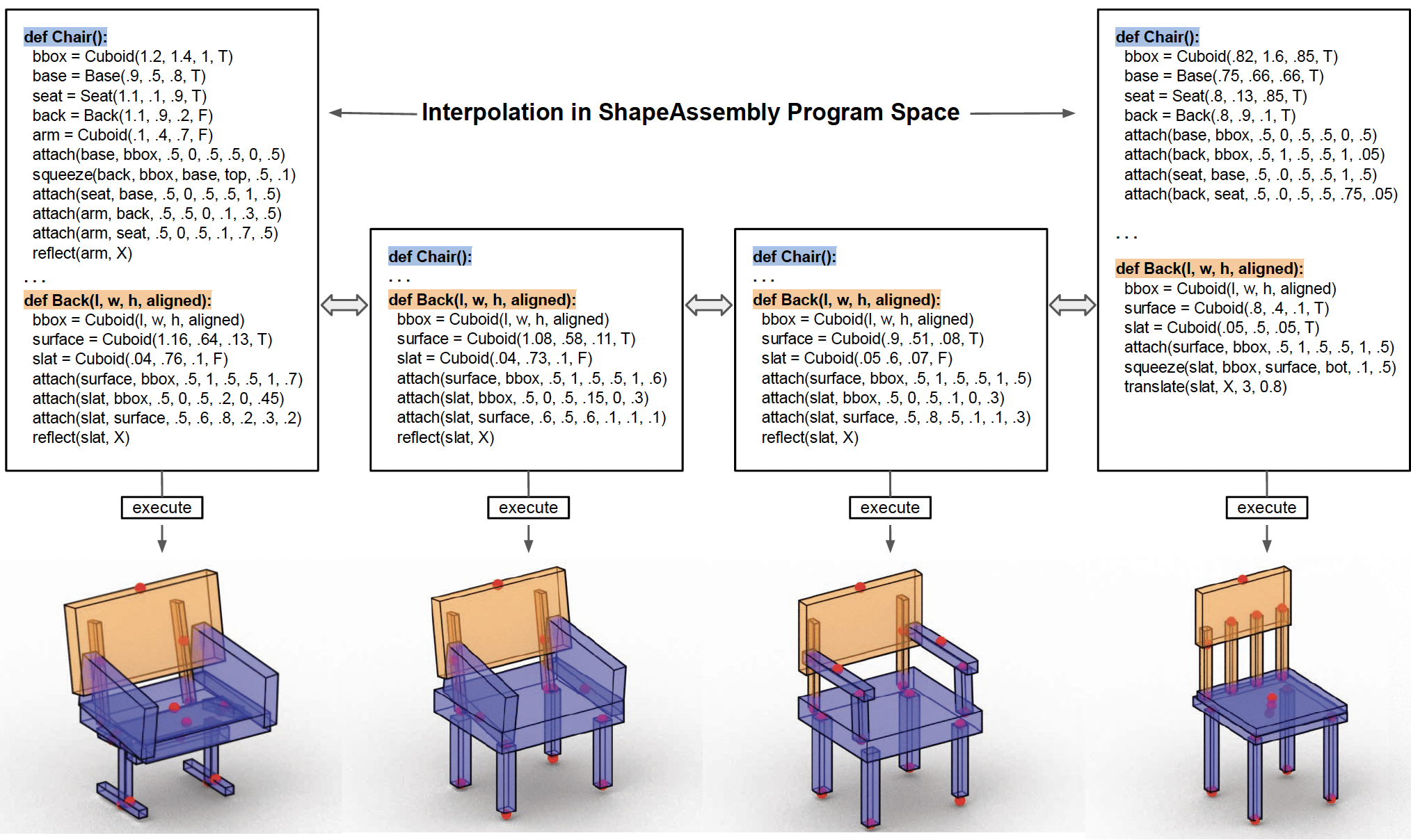
ShapeAssembly: Learning to Generate Programs for 3D Shape Structure Synthesis
SIGGRAPH Asia 2020
Paper
|
Project Page
|
Code
|
Video
|
Supplemental
Using a hybrid neural-procedural approach, we present a deep generative model that learns to synthesize 3D shapes by writing programs in ShapeAssembly, a domain-specific 'assembly language' for 3D shape structures.
Preprints
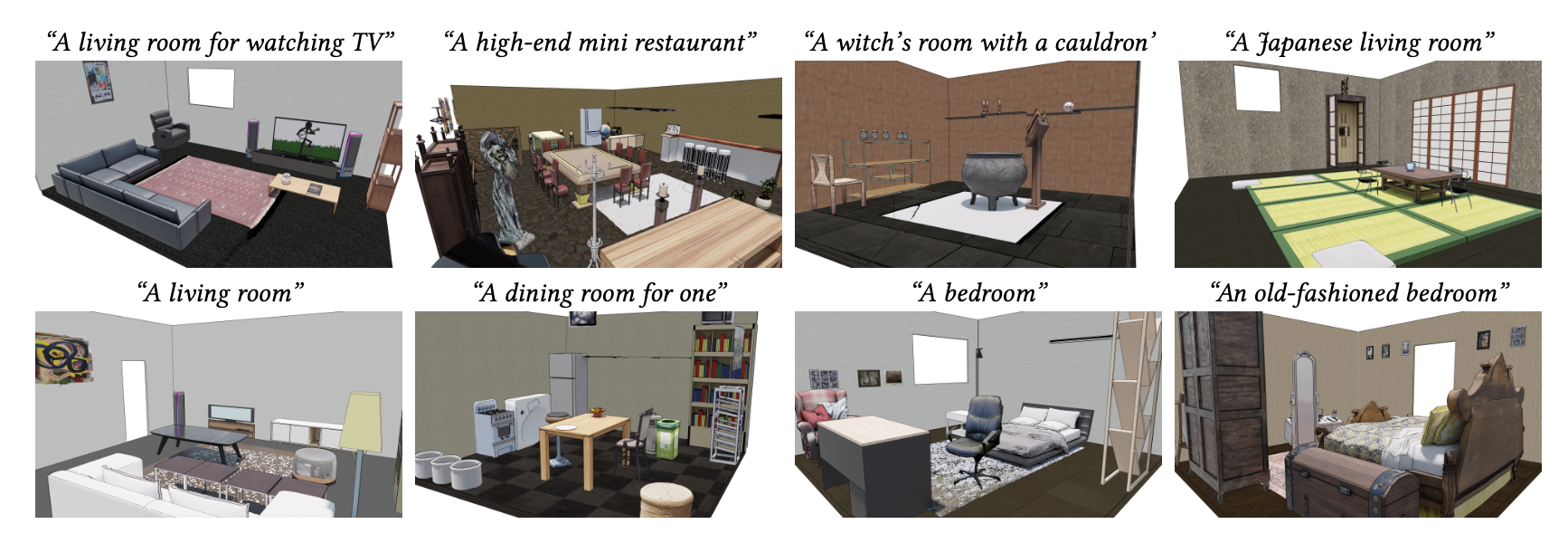
Open-Universe Indoor Scene Generation using LLM Program Synthesis and Uncurated Object Databases
arXiv preprint
Paper
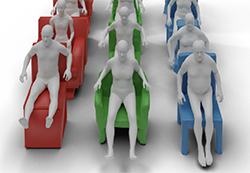